Deciphering the human genome with AI
Prof. Eleftheria Zeggini at the Helmholtz Institute for Translational Genomics researches how risks for complex diseases like diabetes, osteoarthritis, and obesity (the phenotype) can be predicted from the DNA sequence (the genotype). Such prediction based on genomic data can enable early intervention in disease and serve the prevention of complications.
Sequencing the genomes of thousands of healthy and diseased individuals results in vast amounts of data that hold much greater potential than human hands can fully exploit. Computer algorithms, on the other hand, possess this capability. Prof. Zeggini and her team use AI algorithms to identify gene variants in human genomes associated with disease. Furthermore, these algorithms can identify biomarkers that provide prognoses for the course of a disease and identify potential complications. Prof. Zeggini emphasizes that this information is not deterministic but includes a relative risk. Whether a disease actually develops depends on the interplay with various environmental factors.
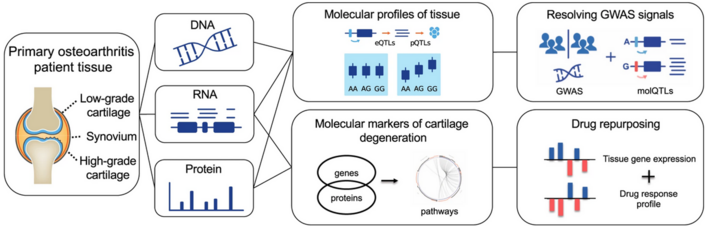
According to Zeggini, the combination of AI and genomic data has proven to be a very helpful tool in analyzing and repurposing existing medications for different medical conditions. The significant potential of this approach is that medications can be deployed more quickly, if shown to be efficacious, since they have already been tested for safety.
Using AI to diagnose and treat genetic diseases
Mutations in DNA can lead to cancer and hereditary diseases, with devastating consequences. The human genome is so vast and complex (3 billion base pairs!) that finding disease-causing mutations is a challenge. Often, this involves just a single mutated base pair. Hence, the genetic causes of cancer and hereditary diseases often remain unclear, alongside potential therapeutic approaches. AI can help to make precise predictions from extensive sequence data – Prof. Julien Gagneur and his research group at the chair for Computational Molecular Medicine at TUM are developing algorithms that predict which mutations lead to faulty gene products and why.
They are particularly interested in so-called non-coding DNA sequences, which constitute 98-99% of the genome. These sequences are not themselves translated into proteins but play a crucial role as regulators of the expression of protein-coding genes. Prof. Gagneur uses advanced AI techniques and vast genomic data to understand the function of these much less explored sequences that make up the majority of the genome.
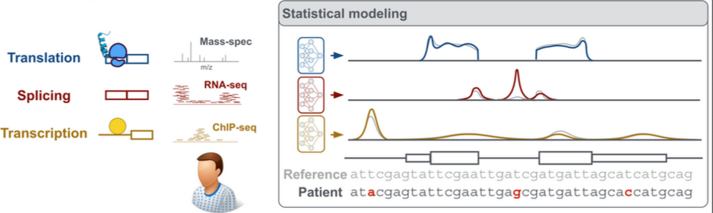
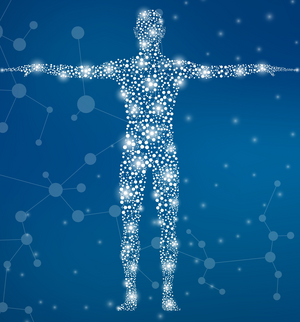
From a single fertilized egg emerges a human being composed of a myriad of distinct cell types – a true masterpiece of nature.
Prof. Fabian Theis and his team at the Helmholtz Munich Computational Health Center are dedicated to unraveling the molecular and biochemical processes that orchestrate this remarkable development. Simultaneously, they investigate the aberrations that occur in patients afflicted with illness.
To address these questions, they sequence and characterize individual body cells, generating substantial volumes of Big Data. In order to unearth answers from this data deluge, they craft AI algorithms, employing a method known as unsupervised machine learning. This type of AI analyses data without a specific query and classifies cell types based on commonalities, such as a similar gene expression profile. The more akin cells are, the closer they align within a biological developmental process.
Through this methodology, AI constructs models that illustrate the developmental stages of cell types and tissues. Drawing from these cellular atlases of human organs, biochemical markers can be identified and applied for diagnosis, including the identification of metabolic diseases. Furthermore, these cellular atlases prove invaluable in developing medications with enhanced precision, focusing on very specific processes and reducing the likelihood of side effects.