Munich's expertise in applying AI to research and medicine
In Munich, medical research institutions are concentrated like few other places in Germany. The TUM and LMU with their university hospitals, Helmholtz Munich and the Fraunhofer Institute are pioneers in researching AI applications in medicine. In this context, the topics of data security and data-processing law must not be neglected. Here, we present just a few selected experts.
![[Translate to English:] Helmholtz Munich Banner](/fileadmin/_processed_/5/4/csm_Helmholtz_Munich_Pforte_with_new_corporate_design_3532c0d15d.jpg)
Cell atlasses
The industry often refers to Big Data as the "oil of the future" because Big Data allows us to comprehend and control our health. Prof. Fabian Theis and his team leverage genomic and biochemical data to investigate the intricacies of cellular processes and how errors can lead to the onset of disease.
Professor Theis is at the forefront of developing unsupervised machine learning algorithms that utilize sequencing data from individual body cells to construct models of both healthy and diseased organs. Much like a map provides a geographical description of the world, these models elucidate the biology of human cells and tissues. They empower us to determine the when and why behind the emergence of diseases and explore innovative treatment possibilities.
→ More about Fabian Theis' research
Big Data in translational genomics
Prof. Eleftheria Zeggini unleashes the power of AI to predict disease risk and develop medicines and prevention approaches from genomic data (the sequences and modifications of DNA, RNA, and proteins). To train meaningful AI algorithms, sequences from hundreds of individual genomes are required – the more and the more diverse, the better.
Data protection barriers stand in the way of processing genomic data for research purposes. Therefore, Professor Zeggini is excited about the establishment of the M1 Munich medicine alliance. This initiative aims to pave the way for her and other researchers to collaborate without bureaucratic obstacles, exchange data for research purposes, and conduct standardized clinical studies. This will enable the tremendous potential of genomic big data to be even better harnessed in research and clinical applications.
→ More about Eleftheria Zeggini's research
Medical imaging
In collaboration with radiologists from the University Hospital of the TUM, Prof. Julia Schnabel is pioneering the development of AI algorithms aimed at advancing every facet of medical imaging. Leveraging machine learning, these algorithms assist not only in image acquisition but also in automating image data measurements, ultimately enhancing the precision of diagnoses and prognoses.
By utilizing image data from healthy individuals, AI models are trained to detect anomalies in imaging procedures such as ultrasound, magnetic resonance imaging, and computed tomography. These models operate with such precision that they can diagnose conditions like lung cancer in their earliest stages, thereby enhancing treatment possibilities.
→ More about Julia Schnabel's research
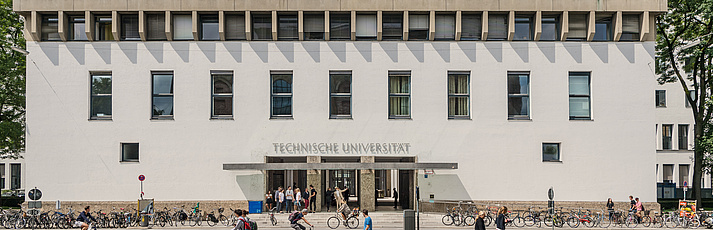
Technical University of Munich (TUM)
-Omics and gene expression
Prof. Julien Gagneur combines -omics analyses (genomics, transcriptomics, proteomics) with AI to understand how cells regulate the expression of the DNA code und thereby their function. This fundamental knowledge will enable the diagnosis and treatment of diseases arising from faulty gene expression.
To also unravel the genetic causes of rare diseases or cancer, which sometimes arise from the mutation of just a single base in a genome of over three billion base pairs, an extensive collection of genomic patient data is required. Therefore, Prof. Gagneur is committed to establishing a cloud that allows the storage and provision of clinical data for research purposes.
→ More about Julien Gagneur's research
Radiology and data security
Prof. Daniel Rückert and his team at the Institute for Artificial Intelligence and Informatics in Medicine work in close proximity to the TUM University Hospital. This proximity to patients and medical professionals is extremely beneficial for the development, adaptation, and translation of AI applications into clinical practice. Prof. Rückert's primary focus is on advancing methods in medical imaging.
Training effective AI algorithms relies on a substantial amount of patient data. To ensure secure data utilization, Prof. Rückert and colleagues develop AI models known as "privacy-preserving AI," ensuring that patient data remains within the hospital. Instead, AI models are trained within the respective hospitals, allowing them to be shared among researchers without exposing patient data.
→ More about Daniel Rückert's research
Constructive data protection
Prof. Dirk Heckmann conducts interdisciplinary research across various domains of digitalization, particularly within healthcare. He pursues an approach centered on constructive data protection to build bridges toward data solidarity.Such an approach aims to strengthen trust between doctors and patients, allowing us to optimally harness the supportive role of AI in advancing human health.
A lack of data exchange in healthcare can have devastating consequences; for example, tens of thousands of people die annually due to drug interactions and side effects that could be prevented through improved information sharing. Prof. Heckmann leverages his legal expertise in interdisciplinary analyses of data security to ensure safety in the path toward digitalization while unlocking the immense potential of AI applications in medicine. In pursuit of this goal, he leads the digital transformation of the healthcare sector as the Managing Director of the Scientific Advisory Board of AOK Nordost.
![[Translate to English:] LMU Banner](/fileadmin/_processed_/c/3/csm_LMU_banner_065ab955d2.png)
Ludwig-Maximilians-University of Munich (LMU)
Cancer research and pathology
Prof. Frederick Klauschen began using machine learning to study cancer during his time at the Charité in Berlin. Since moving to the LMU, he has expanded his efforts to not only employ AI methods in research but also establish them in clinical diagnostics.
Prof. Klauschen also sees significant potential in AI for bridging the gap between biomedical basic research and data-driven clinical research. Explainable AI (XAI) methods can predict mechanistic or causal relationships from complex clinical datasets. Hypotheses can be formed from these predictions and then validated in experiments or further clinical studies. This approach, for example, is intended to assist in the development of personalized therapies for treating cancer.
→ More about Frederick Klauschen's research